Plastic is an essential part of everyday life, from water bottles to packaging to technical molded parts for specialized equipment. With its inherent durability and resistance to water, it is one of the most common materials used today.
Assistant professor in the Department of Mechanical Engineering at the University of Connecticut, Ying Li has received a prestigious Young Investigator Award from the U.S. Air Force Office of Scientific Research to develop the next generation of cutting-edge thermoset plastics using artificial intelligence technology. As part of the award, Li will receive $450,000 from AFOSR to conduct his research.
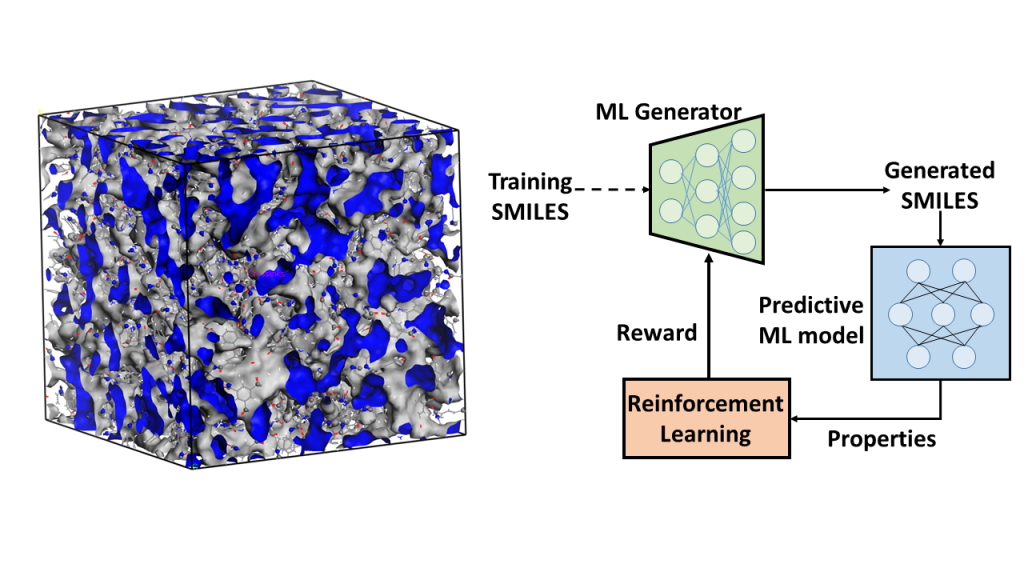
Thermosetting polymers, or thermoset plastics, are different from traditional plastics because they become irreversibly rigid when heated. Once hardened, thermoset plastic products can withstand high temperatures without losing their structure, making them ideal for high-heat applications like electronics and appliances. In fact, many common plastics are thermosets including epoxies, silicones, polyesters, and vulcanized rubber which is used in tires.
The development of thermosetting polymers such as epoxies, found in paints and adhesives, has used a trial and error approach. Although this has garnered valid results, it is costly, slow, biased toward certain molecules and limited to relatively small-scale studies. Due to these flaws, potential successful compounds can be overlooked.
Additionally, due to the challenges associated with searching the broad design space with an infinite number of molecular combinations, technology has a ways to go before it can properly automate these processes.
Li plans to address these complex issues using artificial intelligence. He will formulate a deep reinforcement learning approach, which involves combining artificial neural networks with a reinforcement learning structure, thereby enabling the software to learn the best possible ways to discover novel, yet predictable combinations of properties for cutting-edge thermosetting polymers.
This complicated process requires the use of high-throughput molecular simulations, machine learning techniques, and the development of an ‘on-demand’ Bayesian optimization approach. The Bayesian optimization approach is a powerful technique commonly used to update probabilities of a hypothesis as more information becomes available. Combined with deep reinforcement learning, it can open many possibilities to explore a design space that guides the system to effective and adaptive data collection of thermosetting polymers.
Experimentally validated molecular dynamics simulations will provide a deeper understanding of these polymers, especially different epoxies.
Machine learning is an application of artificial intelligence that makes it possible for systems to make accurate predictions without being explicitly programmed. This has the potential to explore the structural and synthetic relationships among thermosetting polymers.
If successful, this research will provide wide-reaching benefits to the scientific community by addressing an array of important questions concerning computational materials design, microstructure characterization, inverse molecular design, and the synthesis-structure-property relationship.
This research will help the broader technical community and defense industries to develop new types of thermosetting polymers with improved and customized thermomechanical properties. These new polymers could show great promise for aerospace applications due to their superior processability by cost-effective composite manufacturing techniques and higher temperature performance.
Ying Li received his M.S. in engineering mechanics from Tsinghua University in 2008 and his Ph.D. in mechanical engineering from Northwestern University in 2015. Li’s research interests include multiscale modeling of biological system, computational materials design, mechanics and physics of soft matter, machine learning-guided polymer design. Li was awarded the 3M Non-Tenured Faculty Award in 2020, ASME Haythornthwaite Young Investigator Award in 2019, and the International Institute for Nanotechnology Outstanding Researcher Award in 2014.