When Anji Seth was in graduate school, she never thought of herself as a big data scientist.
She just went to her engineering and atmospheric science classes, did the computer programming that was required, and learned as she went.
“All of my classes required some kind of programming – it was a natural thing,” she notes. “But we didn’t train specifically on it – we just did it. Climate science is one of the original ‘big data’ problems, but we didn’t always call it that.”
Now, as a professor in UConn’s department of geography, she still doesn’t refer to herself a data scientist – she’s a climate scientist, first and foremost, she says. But, she notes, that’s the beauty of data science: it’s a “big umbrella,” she says.
Seth is one of many scientists, social scientists, and even humanists across the College of Liberal Arts and Sciences whose work overlaps the realm of big data, a major component of the College’s research portfolio.
Their work is inherently interdisciplinary, team-focused, and constantly changing.
“Our work gets more and more complicated and computationally intensive over time,” says Seth. “So the data is inherently big, and getting bigger.”
Climate Challenge
For a place like Connecticut, with a relatively small geographic area, Seth’s climate modeling work takes on special significance.
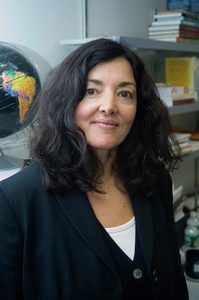
Climate model projections are done globally, using computer models of climate that simulate temperature, wind speed, precipitation, humidity, and dozens of other variables at regular intervals around the globe. The areas between simulated points, called grid cells, can be very large in size.
“Connecticut is only a few grid cells,” Seth points out. “So how can we have confidence in detailed projections of climate change effects for the state of Connecticut?”
She says there are ways to analyze multiple climate models to provide more detailed data for smaller geographic scales, but running a single global model at a resolution of 10 kilometers per cell – instead of the usual 100 — requires an enormous amount of computer time.
In addition to their own high-powered computers, she and her graduate students use UConn’s High Performance Computing facilities for their work. This centralized computing facility has more than 11,000 cores – each comparable to a traditional computer – and more than 200 data analysis programs for researcher use.
Working with Governor Ned Lamont’s Climate Change Council (GC3), and using the data analysis methods she’s developed for her own research, Seth co-led a 2019 effort to produce a state climate change report. The GC3 report presented the results to Lamont in January 2021.
The GC3 report spurred the development of three pieces of state legislation concerning transportation cap and trade systems, climate adaptation and reducing greenhouse gases. The first of these passed out of committee and will be heard at the legislative session in the coming weeks.
At UConn, Seth has worked for a year and a half on the UConn President’s Working Group on Sustainability and the Environment, an internal working group concerned with transforming UConn to a zero-carbon campus. The working group is a response to student protest surrounding the Fridays for the Future movement that climate activist Greta Thunberg began in 2018.
The committee made several recommendations to the President and the Board of Trustees in April 2021, on a path forward toward zero-carbon.
“I am steeped in the climate science, so I can give you all the reasons why this is so urgent,” she says. “We must aim for zero [carbon emissions] by 2040. The science requires it. Environmental justice requires it. As a public university sustainability leader, we can help the state and the nation meet our commitments to the Paris Agreement.”
Inner Space
Every year, a wealth of new questions arise about what is happening in outer space, says astronomer Cara Battersby. And each of those questions requires more data and more computing.
“As our understanding of the Universe becomes more sophisticated, the questions we can ask become more complex, with each generation needing more and more data to ask the next big questions,” the assistant professor of physics says
Battersby’s work focuses on describing and studying the center of the Milky Way galaxy, which she calls an “experimental playground” for the distant cosmos.
She studies this area because it has properties similar to faraway galaxies, and can help us understand cosmic occurrences that would otherwise be more difficult to study.
“It’s denser, hotter and at a higher pressure than the rest of our galaxy,” she says.
Battersby works on data from the Submillimeter Array facility, a collection of eight powerful telescopes situated atop Mount Maunakea, the highest point in Hawaii. The telescope can collect up to a terabyte of data every day, and Battersby’s project used 61 days of data.
Her work described the spectroscopy of the galaxy’s center, which analyzes imagery of the area to understand the chemical makeup of the area, as well as its temperature and the velocity of objects.
Importantly, she says, we can then compare these descriptors to other areas of the universe, to determine their similarities and understand how processes will work within them.
“Previous models were formed using information from the disc of the galaxy,” she says, where physical properties are very different from the center. “Our survey is the first to be sensitive to all these star-forming cores.”
The star-forming cores, or precursors to stars, have turned out to produce stars about 10 times slower than cores in the disc of the galaxy. Battersby says this difference is crucial to getting models right to interpret information gathered from far-off ends of the universe.
Her publications sets the stage for future predictive work done in the center of the galaxy.
Battersby refers to her computer as “her laboratory,” and ensures the students in her classes do, too. In her courses she often assigns programming and analysis problems, like using a large data set to determine the material composition of the Sun.
“We have a lot of the tools to train students in data science,” she says. “Research is moving in that direction, and students in our programs are prepared for it.”
When The Data are Missing
“I was trained as a statistician, to do theoretical and methodological statistics,” he says. “But at the end of the day, I enjoy solving real world problems.”
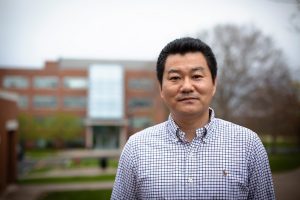
One recent common link among projects on which he’s consulted is that of uncertain, missing or inconsistent data.
In a recent publication, he and colleagues present a new way of approaching large data sets with models to understand what’s called heterogeneity, or how different factors influence the outcome of an analysis in different ways.
For example, an imaging-genetics study may involve hundreds or thousands of possible genetic markers that may influence Alzheimer’s disease, and a predictive model may identify 30 of those as the most useful and important to study. Of those 30 markers, Chen says, his new model can help determine which have what kinds of effects in different subgroups of patients – like, which are active or inactive at different stages of the disease.
This work is in collaboration with researchers at UConn Health, Yale University, and University of California, Riverside.
Chen’s model can also work to understand social questions, such as the risk of suicide among students at particular school districts. Using demographic, socioeconomic and academic data, Chen and Robert Aseltine, professor and chair of behavioral science and community health, have worked with Connecticut schools to study the relative risk of suicide attempts among their student populations.
In this case, the goal isn’t always to match up with reality, he notes. If the actual rate of suicide attempts is higher or lower than predicted by the district-level factors, the “outlying” school could be a subject of future study.
He is also working with Aseltine, a medical sociologist, and Fei Wang at Weill Cornell Medicine, a computer scientist, to determine suicide risks in clinical settings with big medical claims and electronic health records data.
“Can we actually predict the risk of suicide to improve suicide prevention?” he asks. “It’s a fascinating question. If I can do something to help figure it out, that makes me excited.”
Chen also collaborates with Board of Trustees Distinguished Professor of Psychological Sciences Blair Johnson and Professor of Sociology Mary Bernstein to understand community-level factors contributing to rates of gun violence. His new models may be applicable since gun violence data is collected primarily from qualitative sources, such as news reports, so tends to be inconsistent and have missing data points.
Chen enjoys the diversity of research his background allows him to work on, and he sees collaborative work as the future not only of applied statistics, but of big data in general.
“There’s no way I, as a statistician, can do all the work or can even understand the problem as deep as a domain expert,” he says. “If you want to address big questions, you have to have a team of people.”
This article is the final story in a series about emerging research areas in UConn’s College of Liberal Arts and Sciences. Learn more at #DiscoverUConnCLAS.